Today’s technological landscape has been shaped by amazing advances in what we can process, how computers understand us, and what computers can tell us. Users today expect so much more from technology than ever before. We expect technology to connect and give us ubiquitous, current information. Moreover, we expect it to adjust to our specific user profile, tell us about the future of unknown events, and guide us in our decision-making. Some examples include forecasting the weather, user purchasing preferences, or even stock price fluctuations. The solution to these and many other tasks come from predictive analytics.
What’s Predictive Analytics?
In the field of data mining, predictive analytics encompasses the techniques used to predict trends and outcome patterns based on extracted data. This data could be current or historical data pertinent to the outcome we are trying to predict. It can be extracted internally to a system or from any source, including the internet. Credit card fraud or loan delinquency, for example, have strong indicators that can be used to detect and predict their occurrence.
The key lies in the strong relationship between the outcome and the extracted past data. Techniques such as principle component analysis and factor analysis can help determine which pieces of past data are most relevant in explaining an observation and which can be ignored. For instance, we can determine that payment history can determine loan delinquency quite reliably, but other indicators like age or location may provide much weaker links.
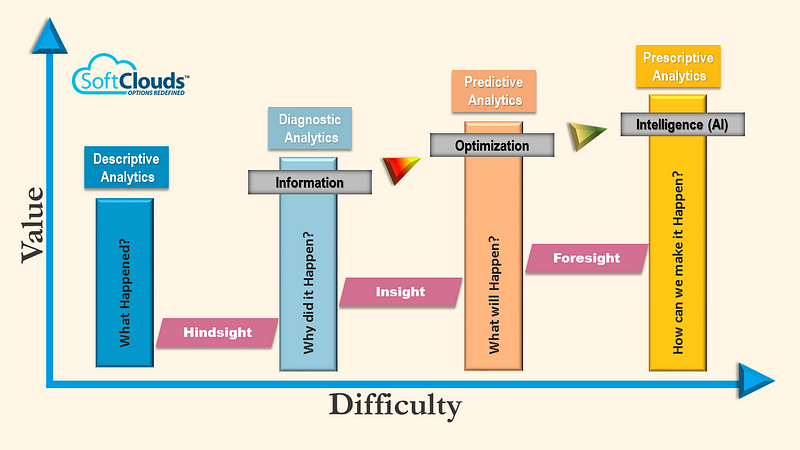
What problems can Predictive Analytics Solve?
What is the weather going to be like tomorrow? What are my most frequent customers going to buy this season? Are my investments low-risk? These are some practical questions for which predictive analytics can provide answers. In any situation where we have information on what we wish to predict, we can use large-scale aggregate data to create models to predict future events. Of course, this works better in some domains as opposed to others.
A longstanding application of these techniques is in credit risk management. Customer payment history, loan requests, debt-to-income ratio, and other factors can predict the likelihood of a customer defaulting on new loans. This information helps loan providers make better decisions to decrease the likelihood of default and optimize their bottom line.
In other domains such as consumer purchasing, we can model consumer behavior and make predictions about future activity from past purchases, product views, or demographic information such as location, age, gender, and so on. Marketers can use this data to create targeted, specific advertisements geared towards the correct customer bases to optimize the likelihood of purchase and increase revenue.

How can this help CRM?
CRM systems today have to address a wide variety of tasks. Users must be able to access information, manage accounts, follow leads, generate new leads and opportunities, and close deals. While CRM platforms like Siebel and Salesforce allow users to perform these tasks, these systems typically only allow access to information that’s current or past information in the system. These systems don’t provide users with a plethora of more information that is predictive in nature and might help them perform CRM-related functions.
For example, with predictive analytics, we can determine which sales opportunities are most likely to close based on revenue, locational proximity, past customer deals, and many other factors. This can guide users of CRM systems to focus on leads that are most likely to generate revenue for the business. All of this is a result of the power of relevant, connected, and accurate predictions.
The second point of interest is delayed sales cycles. Many things could cause delays such as manufacturing delays, sourcing of materials for product creation, scheduling meeting times with clients to move processes along, lack of customer engagement, and so on. Any of a thousand factors can cause these delays. Using predictive analytics we can determine which characteristics of a potential client and your business’s relationship actions are responsible. Armed with this information, businesses can close the gap and address these inefficiencies from within. Thus, predictive analytics can help a business externally and internally.
Finally, attrition is one of the biggest problems businesses face when managing customers. Many factors, such as missed follow-ups on leads, not establishing rapport with the right customer base, not offering relevant product suggestions, or holes in your customer support channels, contribute to this. Using predictive analytics, we can determine what causes customers to make the decision to leave your brand, and your product space, and ultimately cast their economic vote elsewhere. Some factors are weak in determining attrition, while some are strong indicators. Predictive analytics allows a model to be built on understanding what causes this problem so that businesses can improve customer retention and provide better service to their customers.

Future Directions
Predictive analytics is one of many emerging technologies with applications in CRM and other sectors. This powerful technology, which has also been used in various applications in the medical field, travel safety and the automotive and travel industries, enables consumers, businesses, and employees to make more informed decisions. Moreover, it is a reliable tool that helps to improve business relations.
Although predictive analytics is mostly used to analyze consumer behavior, it will be much more diverse and customizable in the near future. The opportunities for predictive analytics, which was once predominantly used by telecoms and finance companies, will continue to grow. As capabilities seek to expand, predicting behavior and analyzing risks will be the standard for many companies to have a competitive advantage.
Has your company or any company you work with used predictive analytics to improve their sales process or success rate? If so, we’d love to hear about your experience. If not, how do you see that predictive analytics could help you achieve better sales results?